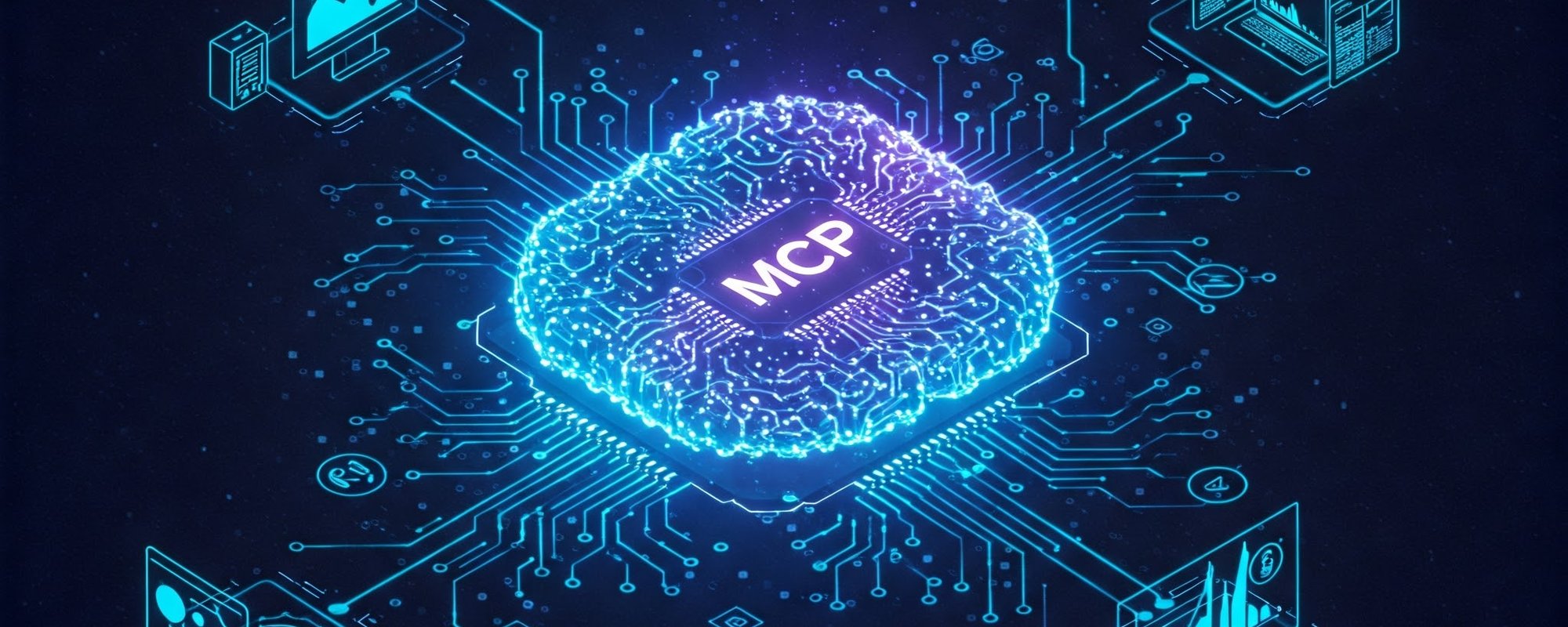
Model Context Protocol (MCP): The Future of AI Integration
In the rapidly evolving landscape of artificial intelligence, a new standard is emerging that promises to revolutionize how we build and deploy AI applications. The Model Context Protocol (MCP) represents a fundamental shift in AI integration architecture, moving beyond traditional API approaches to enable more dynamic, context-aware AI systems.
What is Model Context Protocol?
Model Context Protocol (MCP) is an open standard designed to seamlessly connect AI models with data sources and tools in real-time. Released by Anthropic in November 2024, it has recently gained significant attention among developers and AI practitioners for good reason.
Unlike traditional API-based approaches where AI models function as stateless services, MCP structures AI models as long-lived, context-aware components that can dynamically adjust to user interactions and maintain state throughout a session.
Why MCP is a Game-Changer
Beyond the API Paradigm
For years, we've relied on APIs as the primary method for integrating AI capabilities into our applications. While convenient, this approach has inherent limitations:
- Statelessness: Traditional APIs don't maintain context between requests
- Limited Context: Each request operates in isolation, without awareness of past interactions
- Integration Complexity: Building robust AI systems often requires complex orchestration of multiple API calls
- Latency Challenges: Each API call introduces potential delays in user experience
- Cost Inefficiencies: Redundant processing and constant model reloading increase operational costs
MCP addresses these challenges by establishing a standardized protocol that enables persistent, bidirectional communication between AI models and the applications they power.
Key Benefits of MCP
- Persistent Context: Models retain knowledge across interactions without requiring external memory engineering
- Real-Time Adaptation: AI systems can adjust on-the-fly based on continuous inputs and feedback
- Reduced Latency: By maintaining models in memory rather than reloading them per request
- Standardized Integration: One protocol replaces countless custom APIs
- Dynamic Tool Discovery: AI systems can automatically detect and connect to available tools
- Enhanced Security: Built with strong security controls across AI applications
Real-World Applications of MCP
The potential applications for MCP span virtually every industry:
- Customer Service: Chatbots that truly remember conversation history and context
- Healthcare: AI diagnostics that maintain awareness of patient history
- Education: Personalized learning experiences that adapt continuously to student progress
- Finance: Trading systems and fraud detection with real-time data integration
- Creative Industries: Content generation tools with persistent understanding of creative direction
- Research & Development: AI assistants that maintain context across complex research workflows
Creating an MCP Server: Simpler Than You Think
One of the most exciting aspects of MCP is how it simplifies server creation. Thanks to tools like create-mcp-server
, getting started with MCP is remarkably straightforward.
Quick Start Guide
Setting up an MCP server requires just a few simple commands:
# Using uvx (recommended)
uvx create-mcp-server
# Or using pip
pip install create-mcp-server
create-mcp-server
The tool walks you through the setup process, creating a project structure like this:
my-server/
├── README.md
├── pyproject.toml
└── src/
└── my_server/
├── __init__.py
├── __main__.py
└── server.py
Once installation is complete, starting your server is equally simple:
cd my-server
uv sync --dev --all-extras
uv run my-server
That's it! No complex configuration, no complicated folder structures—just the essentials needed to run your MCP server.
What Makes MCP Server Creation Special?
- Zero Configuration: No need to manually set up project structure or dependencies
- Best Practices: Follows Python packaging standards and MCP server patterns automatically
- Batteries Included: Comes with everything needed to build a functional MCP server
- Simplified Integration: Ready to connect with AI models and data sources
The Growing MCP Ecosystem
MCP is gaining traction rapidly, with adoption by tools like Windsurf, Zed, Replit, and others. This momentum is creating a robust ecosystem of connectors and integrations that make AI deployment more accessible than ever.
As more organizations recognize the benefits of MCP, we're seeing a transition from isolated, API-based AI services to interconnected, context-aware AI systems that can provide more valuable, personalized experiences.
Looking Ahead: The Future of AI Integration
The shift from API-based models to MCP represents a natural evolution in how we build AI systems. As AI becomes more deeply integrated into our applications and workflows, the need for persistent, context-aware models will only grow.
For developers and organizations looking to stay ahead of the curve, now is the time to explore MCP and consider how this emerging standard might transform your approach to AI integration.
Whether you're building customer-facing applications, internal tools, or complex AI systems, MCP offers a more efficient, effective path to creating the intelligent experiences that users increasingly expect.
Conclusion
Model Context Protocol represents a significant leap forward in AI integration architecture. By enabling persistent, context-aware AI models and standardizing how these models interact with data sources and tools, MCP is poised to become the foundation for the next generation of AI applications.
As we continue to push the boundaries of what's possible with AI, standards like MCP will be essential in making advanced AI capabilities more accessible, scalable, and effective. The future of AI integration is here—and it speaks MCP.
Are you exploring MCP for your AI projects? Share your experiences in the comments below!
# server.py
from mcp import MCPServer, MessageRequest, MessageResponse, ToolExecutionRequest
from mcp.tools import Tool, ToolRegistry
# Define a simple calculator tool
class CalculatorTool(Tool):
name = "calculator"
description = "Perform basic arithmetic operations"
async def execute(self, request: ToolExecutionRequest) -> dict:
operation = request.params.get("operation")
a = request.params.get("a", 0)
b = request.params.get("b", 0)
result = None
if operation == "add":
result = a + b
elif operation == "subtract":
result = a - b
elif operation == "multiply":
result = a * b
elif operation == "divide":
if b == 0:
return {"error": "Cannot divide by zero"}
result = a / b
else:
return {"error": f"Unknown operation: {operation}"}
return {"result": result}
# Create server with tool registry
class MyMCPServer(MCPServer):
def __init__(self):
super().__init__()
# Register tools
self.tool_registry = ToolRegistry()
self.tool_registry.register(CalculatorTool())
async def handle_message(self, request: MessageRequest) -> MessageResponse:
# For simplicity, we'll just echo back the message with a note
# In a real implementation, you would send the message to an LLM
return MessageResponse(
messages=[
{
"role": "assistant",
"content": f"Echo: {request.messages[-1]['content']}\n\nTools available: calculator"
}
]
)
async def get_tools(self) -> list[Tool]:
return self.tool_registry.get_tools()
# For running directly (development)
if __name__ == "__main__":
server = MyMCPServer()
server.start(host="0.0.0.0", port=8000)

Django Developer
Django Developer and DevOps Expert specializing in web applications and cloud infrastructure.